Danny Driess
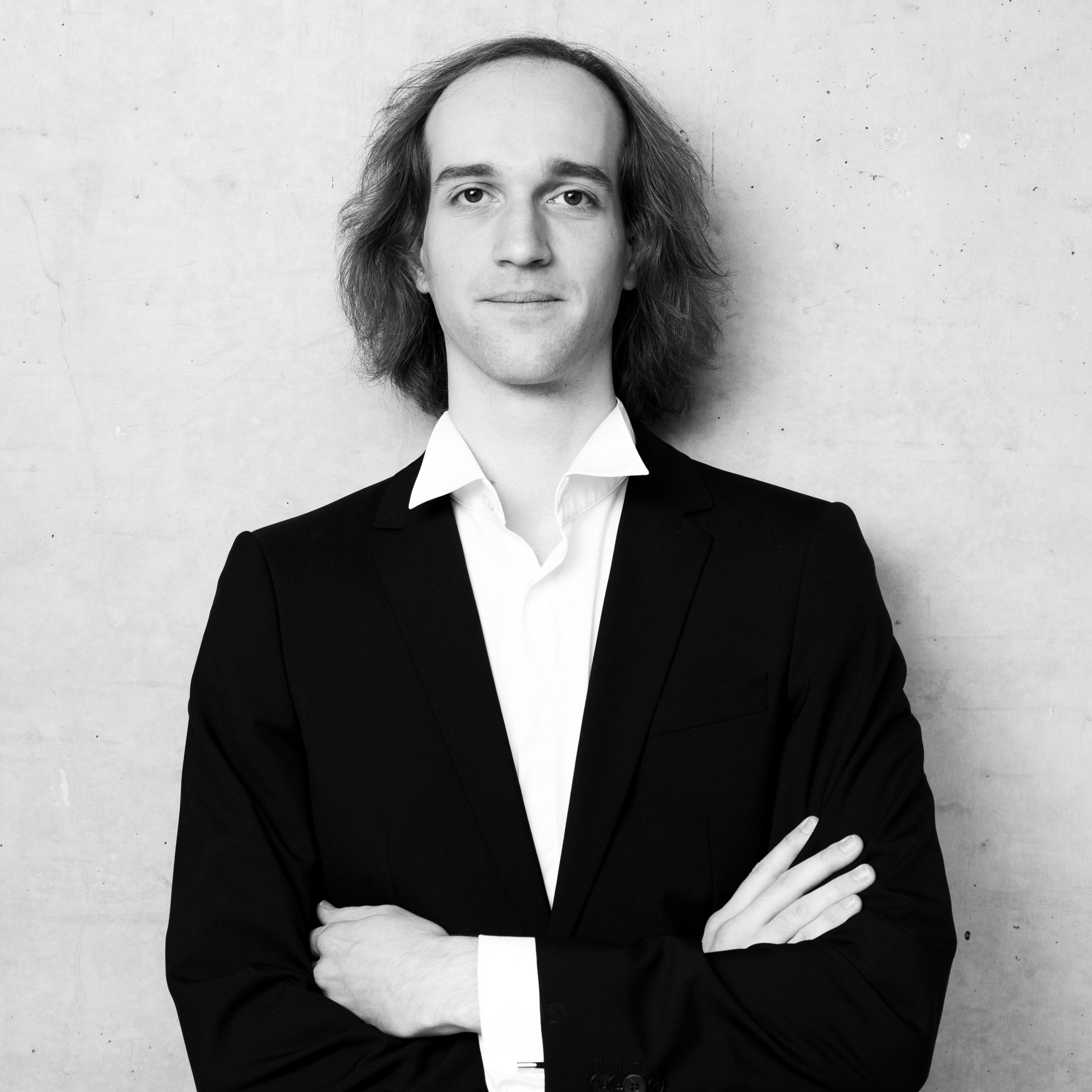
Photo: SCIoI
Danny Driess worked as a doctoral researcher in Marc Toussaint’s team. His PhD focused on learning in Task and Motion Planning with a special focus on connecting perception and planning for sequential manipulation tasks through machine learning. Within SCIoI, he investigated novel ways to couple sequential manipulation planning with reactive policies for execution. He received his Bachelor of Science (with distinction) in Simulation Technology in 2016 and his Master of Science (with distinction) in Simulation Technology in 2019, both from the University of Stuttgart.
Projects
Danny Driess is member of Project 39.
Publications +