Physical manipulation planning with differentiable closed-loop manipulation primitives
Reuniting planning and reacting
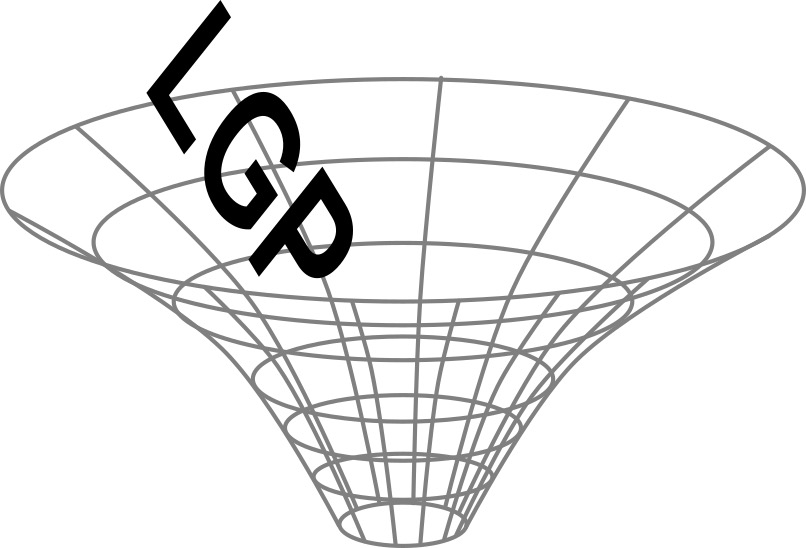
Courtesy of Marc Toussaint
Optimization-based Task and Motion Planning (TAMP) approaches show remarkable capabilities in finding paths given a scene description and (intuitive) physics models. However, the result of a TAMP algorithm is usually an open-loop trajectory, which, when executed in the real world, is likely to fail under disturbances or other sources of
uncertainty. The objective of this project is to bring the generality and computational strength of our TAMP framework to real-world execution. Instead of trying to find controllers that execute a planning result, this project investigates a different, novel approach: Can the behavior of closed-loop (perception-based) control primitives be directly embedded into the planning framework itself such that the result is a sequence of reactive closed-loop control policies instead of open-loop paths? We aim to demonstrate the robustness of our control strategies to severe perturbations, e.g., human interventions, in real-world sequential manipulation tasks such as the escape room scenario.