The collective dynamics underlying personal and social information integration
Understanding how individuals integrate personal and social information
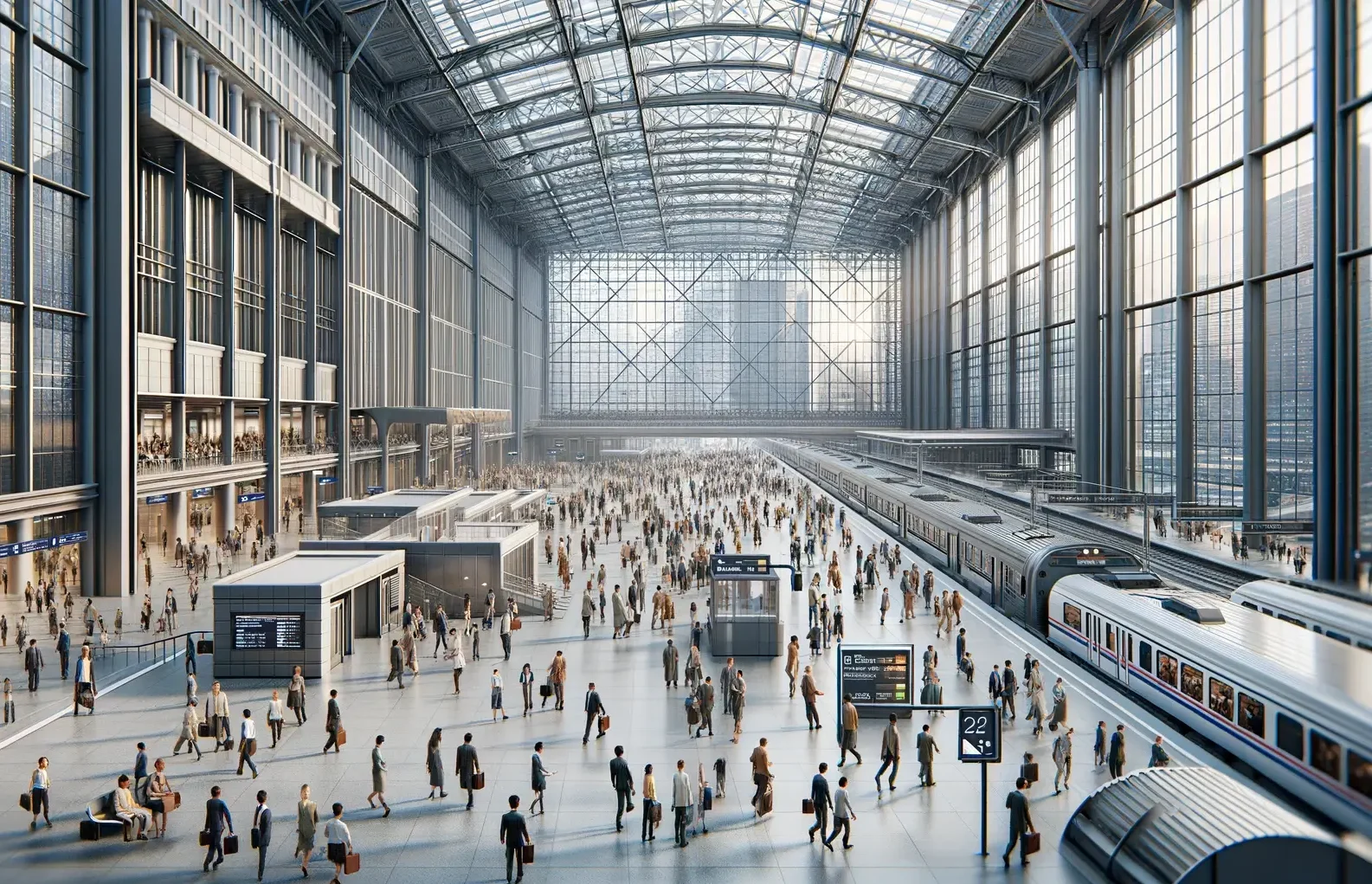
AI-generated at SCIoI with prompt "train station people"
Individuals rarely make decisions in social isolation. In most situations, individuals are subject to social influence. Social influence may be beneficial (e.g., increase decision quality), but it can also be detrimental (e.g., when false information cascades occur). To understand the emergence of collective intelligence—the shared intelligence that emerges from collaborative, collective efforts of individuals—we need to comprehend how individuals integrate personal and social information. A key aspect that has been largely neglected in human collective intelligence research is the dynamic aspect of information exchange. Most studies on human collective decision making assume that individuals simultaneously make decisions, which are then statically exchanged. In reality, however, information exchange is highly dynamic, and the timing of information exchange is linked to (subjective) information quality. Few studies have embraced such an approach; consequently, the dynamics of information flow in human groups remain poorly understood. To fill this important gap, this proposal has four objectives. First, we will investigate how single individuals integrate personal and social information under controlled conditions (objective 1). Next, we will parameterize a dynamic decision making model to predict information flow and collective dynamics in real-time interacting human groups, and test these predictions (objective 2). We then further parameterize our model to derive predictions for information flow across different network structures, and test these predictions (objective 3). Finally, we will bring all these issues together, studying the conditions underlying collective intelligence in collective systems (objective 4). The analytical system consists of human groups conducting experimental choice tasks. The synthetic component consists of drift diffusion models that address the cognitive processes underlying information integration. We continuously close the loop between analytical and synthetic systems, by using both approaches in concert. As end product, we will develop a versatile set of open-source algorithms (in CRAN R/Python) that can be used to study information integration processes in collectives, as well as for programming robotic swarms to achieve collective intelligence in the face of key challenges, such as speed-accuracy trade-offs or optimization at the individual versus collective level. Prior to release, the performance of these algorithms will be extensively tested with genetic algorithms.